Blog
Introducing g6R 0.1.0: a network widget for R.
Create stunning network experiences powered by the 'G6' graph visualisation engine 'JavaScript' library . g6R brings 20 layouts, 15 customisable behaviors like interaxtive edge creation and 17 plugins to improve the user experience such as toolbars. In 'shiny' mode, modify your graph directly from the server function to dynamically interact with the individual components.
Read more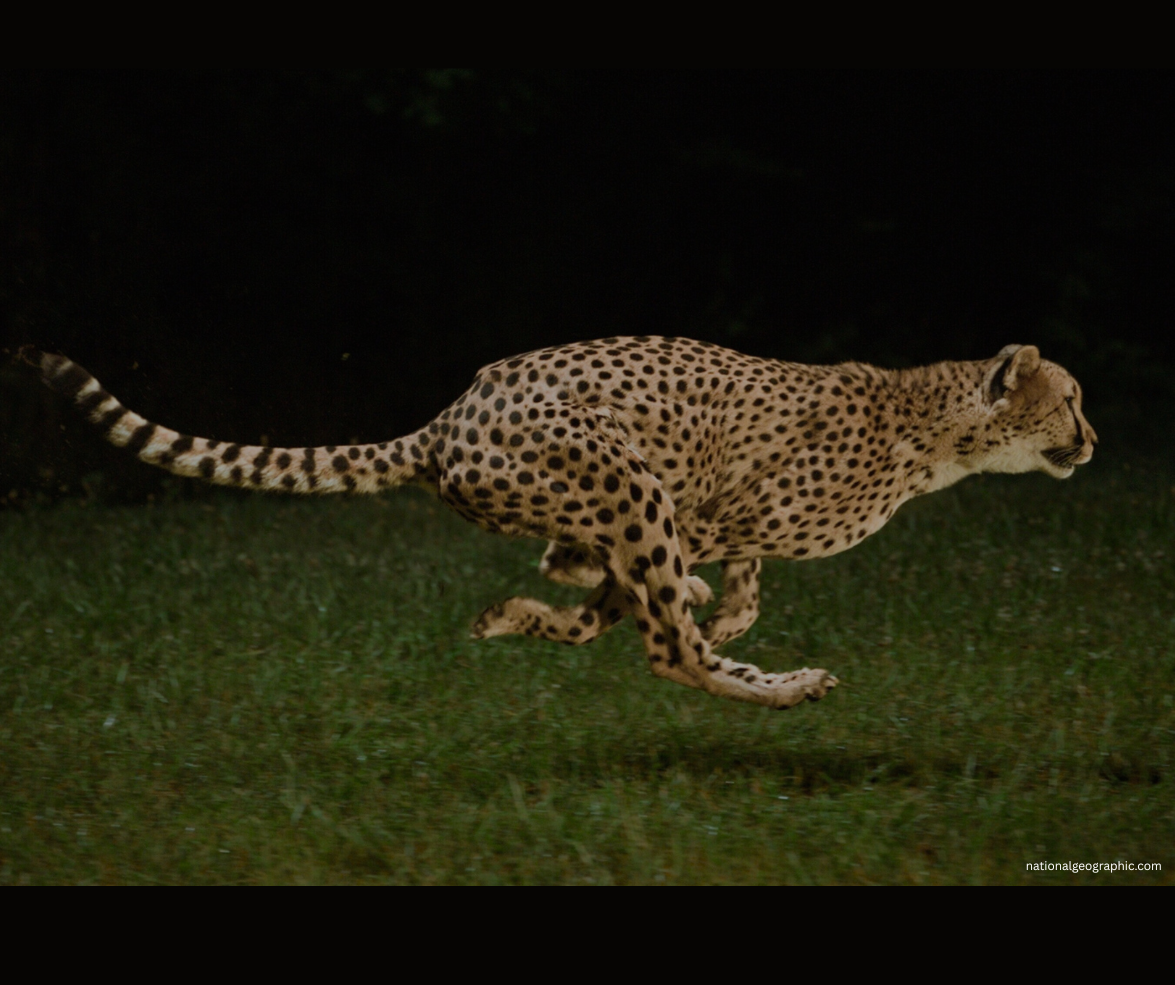
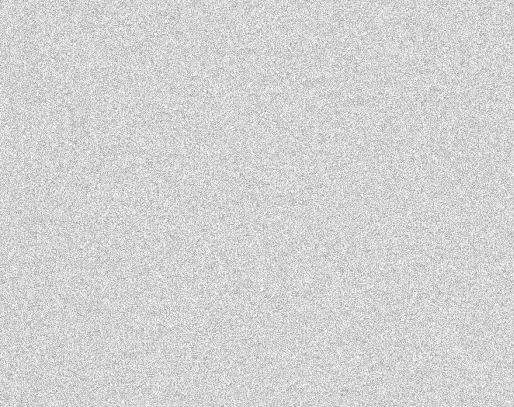
Olajoke Oladipo /
Introducing cheetahR: A Lightning-Fast HTML Table widget for R
R/Tables/htmlWidgets
Say hello to cheetahR — the fastest way to explore massive datasets in R. Now available on CRAN, cheetahR is a high-performance table widget and a modern alternative to {reactable} and {DT}. Built for speed, it lets you interactively explore large datasets with smooth rendering and fully customizable layouts. Plus, it integrates seamlessly with Shiny, making it perfect for powerful, data-heavy dashboards.
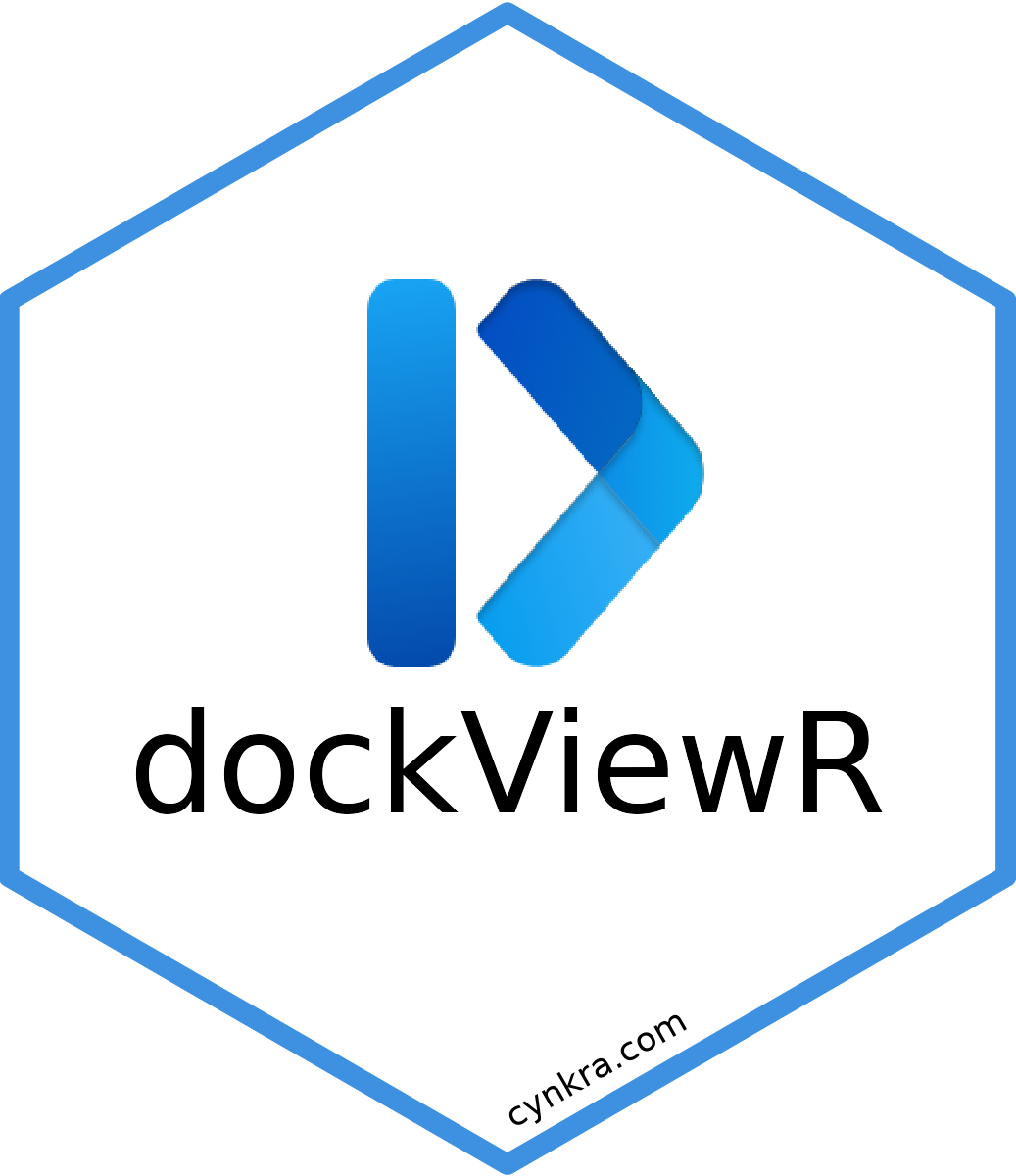
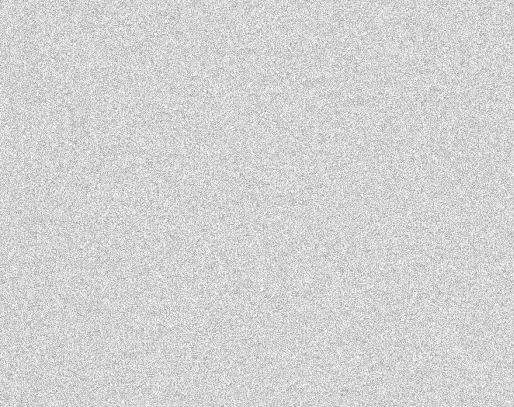
David Granjon, Nelson Stevens, Nicolas Bennett /
Introducing dockViewR 0.1.0: a layout manager for R and Shiny.
Shiny/R
Create fully customizable grid layouts (docks) in seconds to include in interactive R reports with R Markdown or 'Quarto' or in 'shiny' apps . In 'shiny' mode, modify docks by dynamically adding, removing or moving panels or groups of panels from the server function. Choose among 8 stunning themes (dark and light), serialise the state of a dock to restore it later..
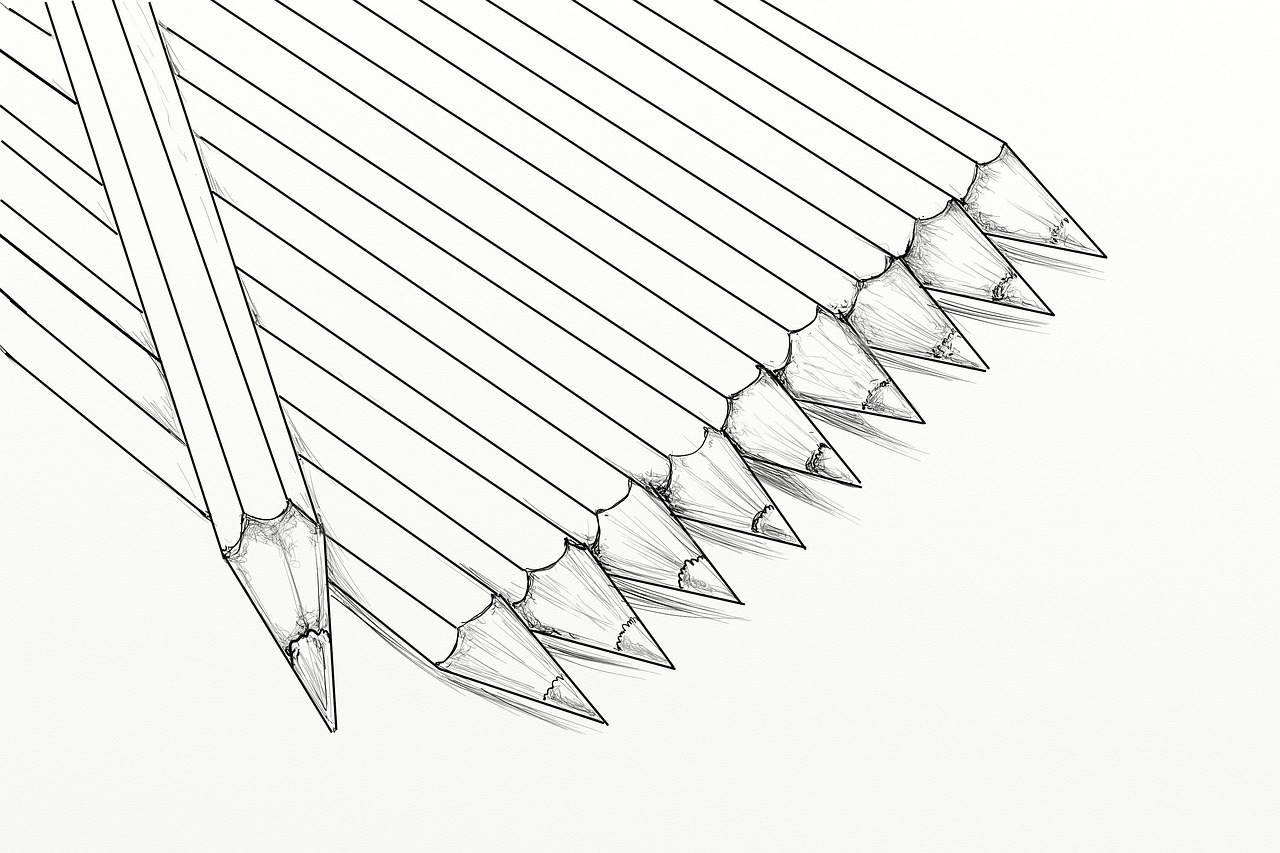
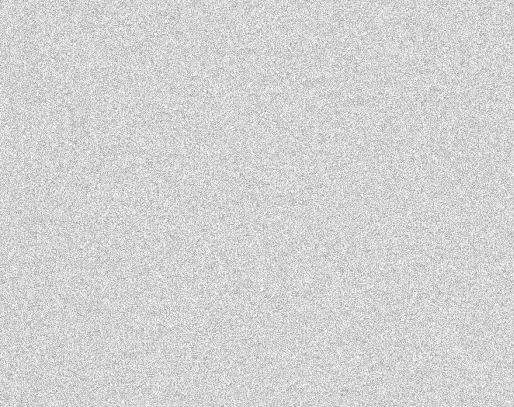
Mike Page /
Introducing shinydraw: a no-code tool for shiny wireframing
Shiny/R
Streamline your Shiny app design: Introducing shinydraw for effortless wireframing
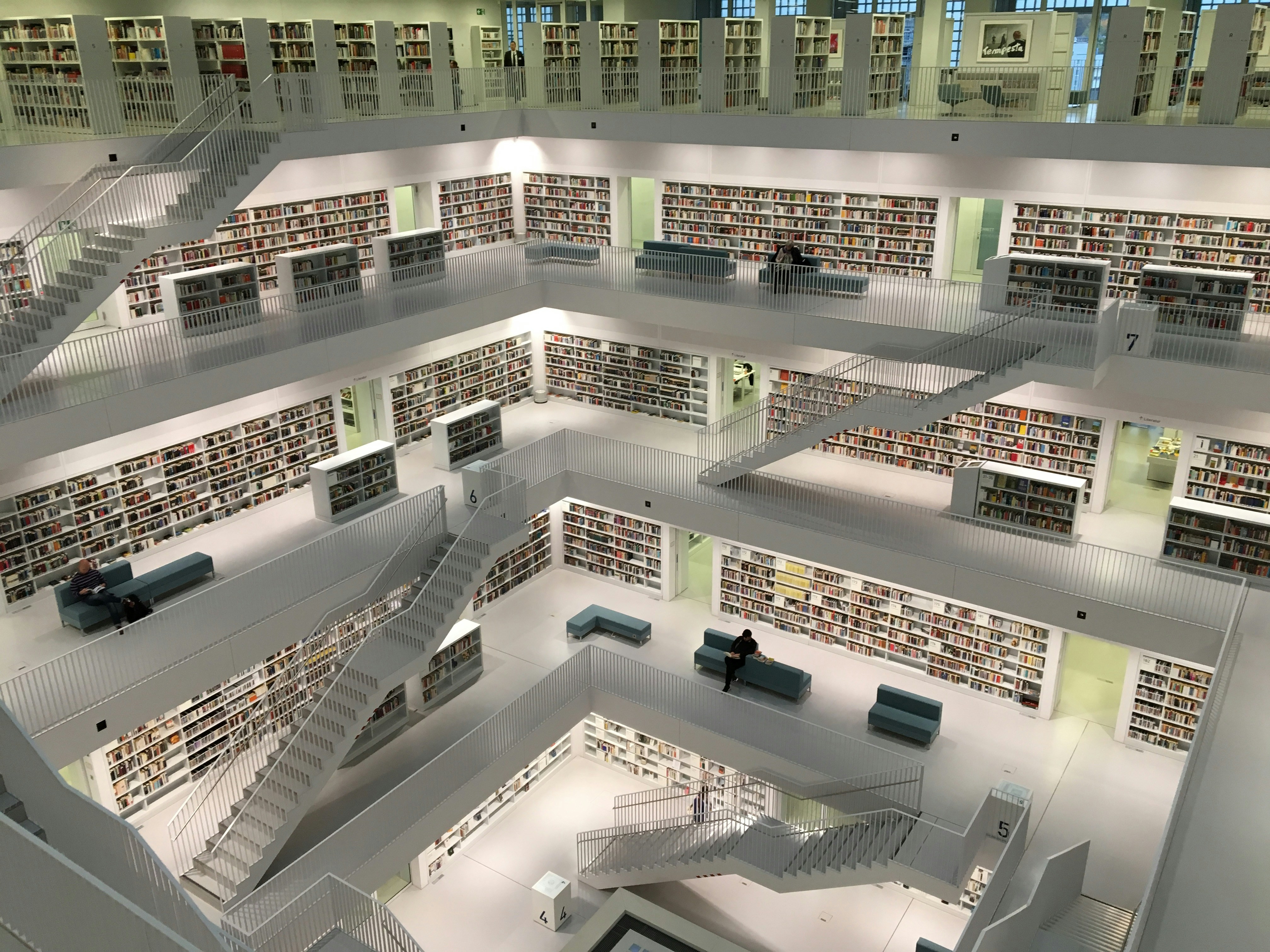
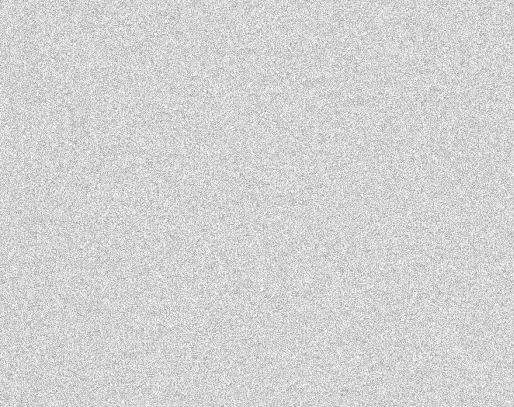
David Schoch, Christoph Sax /
R with RAGS: An Introduction to rchroma and ChromaDB
LLM/RAG/R
Large language models (LLMs) are developing rapidly, but they often lack real-time, specific information. Retrieval-augmented generation (RAG) addresses this by letting LLMs fetch relevant documents during text generation, instead of just using their internal—and potentially outdated— knowledge.
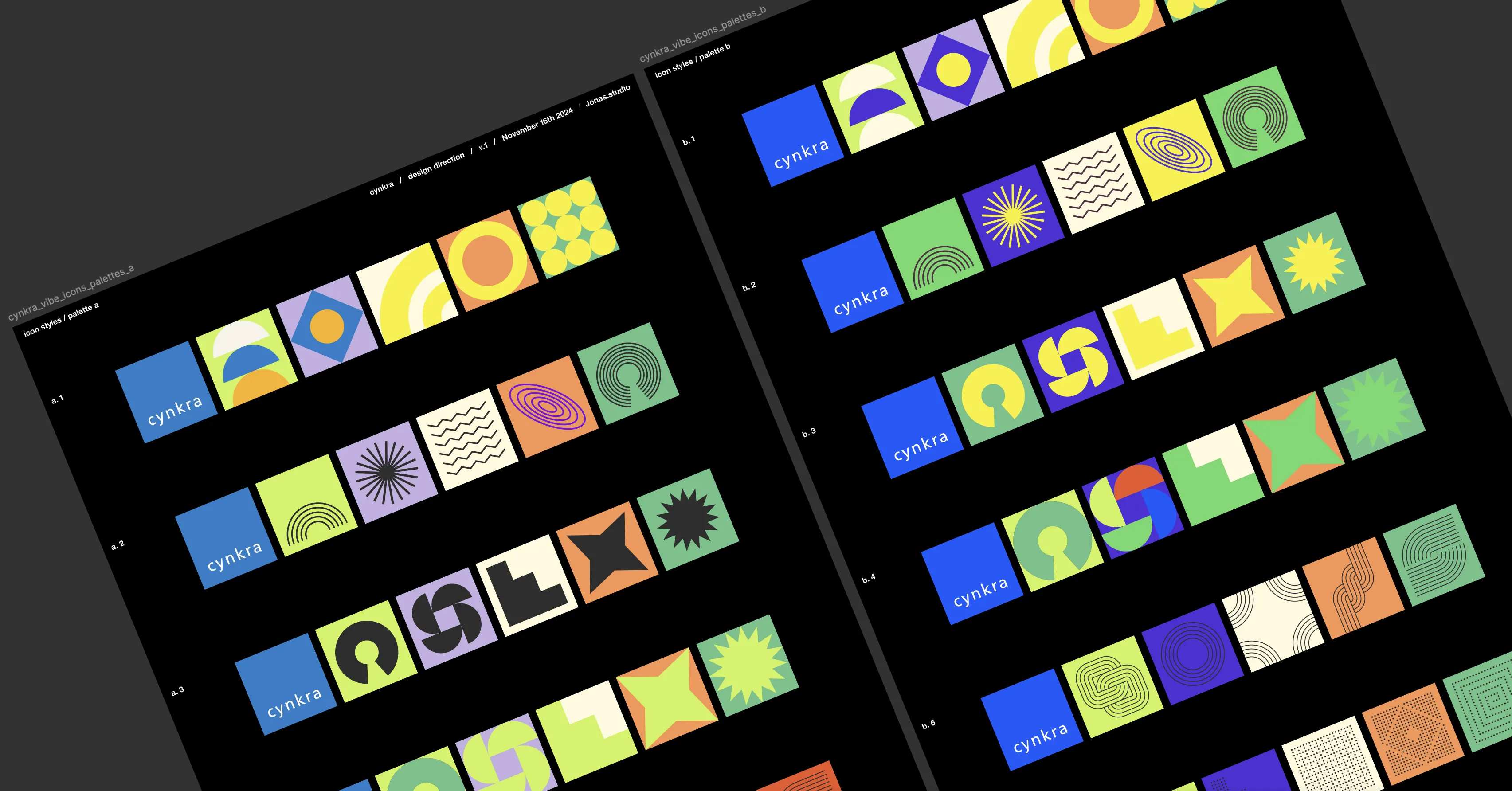
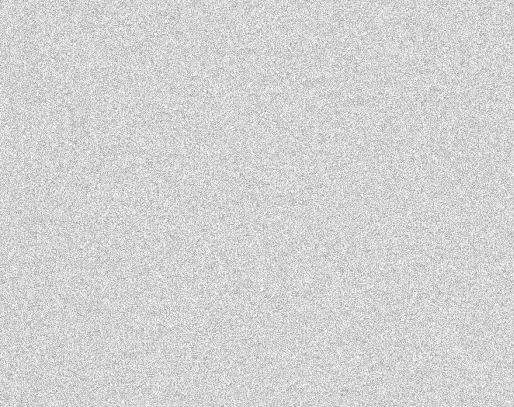
Tamás Tóth, Jonas Goldstein, Christoph Sax /
Website Relaunch
We're thrilled to announce the launch of our completely redesigned website! After months of careful planning and development, we've created a new digital home that embodies our core values: minimalism, functionalism, and clarity.
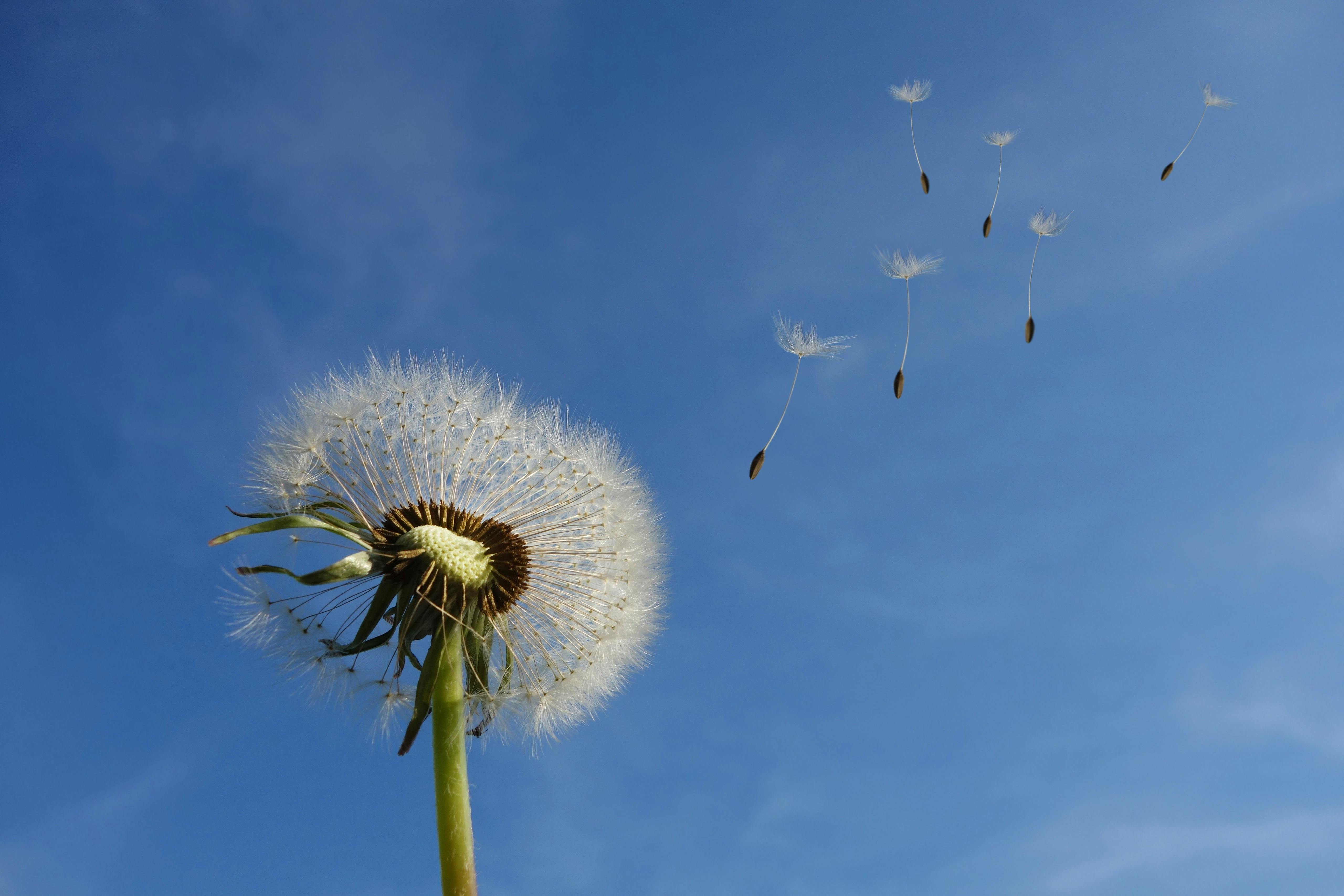
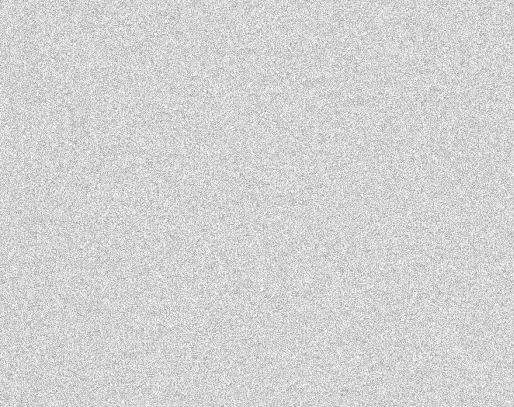
Maëlle Salmon, David Schoch, Kirill Müller /
Setting up igraph for success in the next decade
igraph/R
One year ago, a small group of us at cynkra submitted a project proposal to the R Consortium's ISC, which got approved. We are very grateful for this support. In this post we shall explain what the motivation for our project was, what we accomplished... and what we hope to work on next!
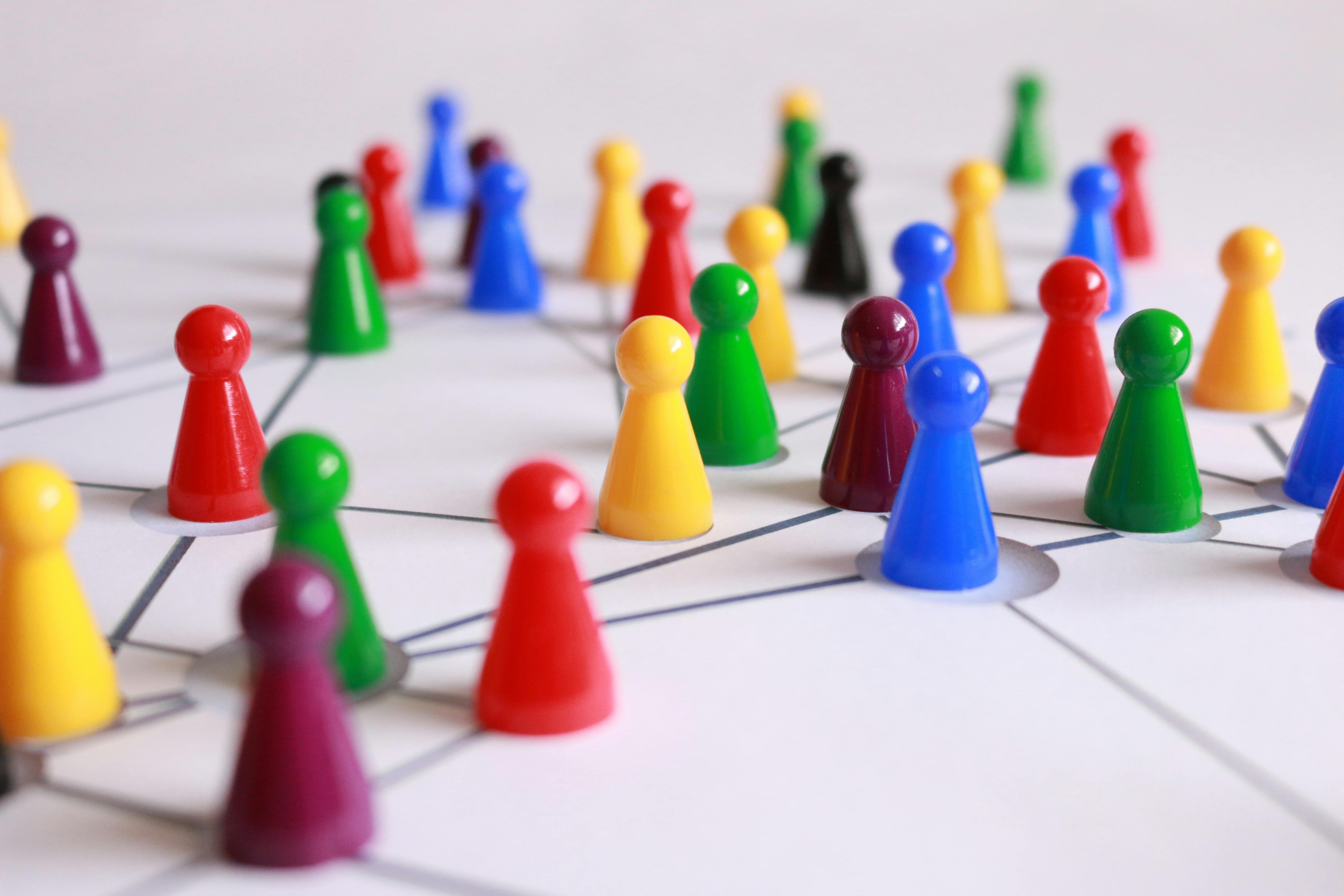
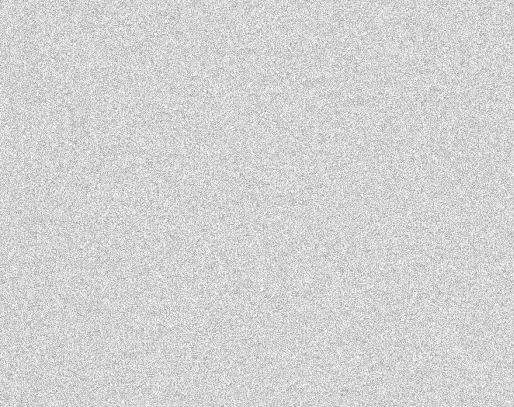
David Schoch /
Organizing tests in R packages
Testing/R
A small team at cynkra is working on the R package igraph, a popular package for simple graphs and network analysis. A big part of this work involves refactoring legacy code and enhancing the maintainability of the package for the future.
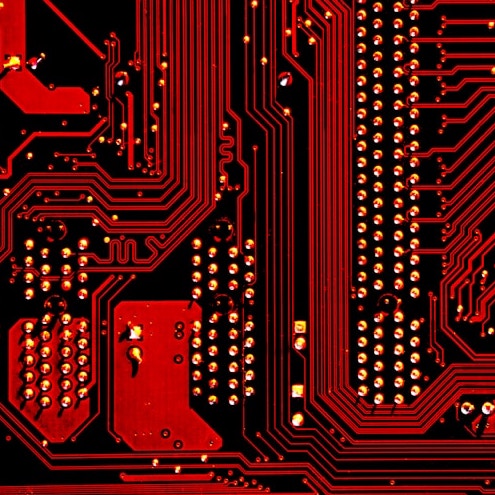
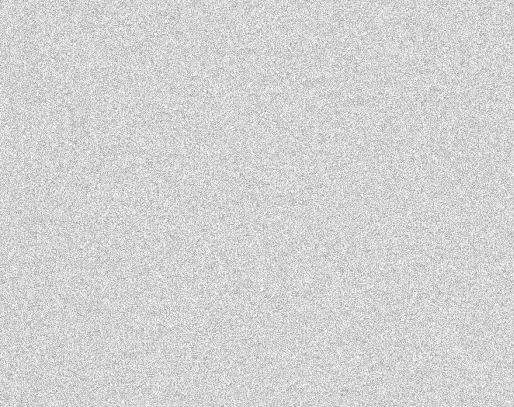
Christoph Sax /
Playing with AI Agents in R
LLM/R
It's local LLM time! What an adventure it has been since I first started exploring local LLMs. With the introduction of various new Llama models, we now have impressive small and large models that run seamlessly on consumer hardware.
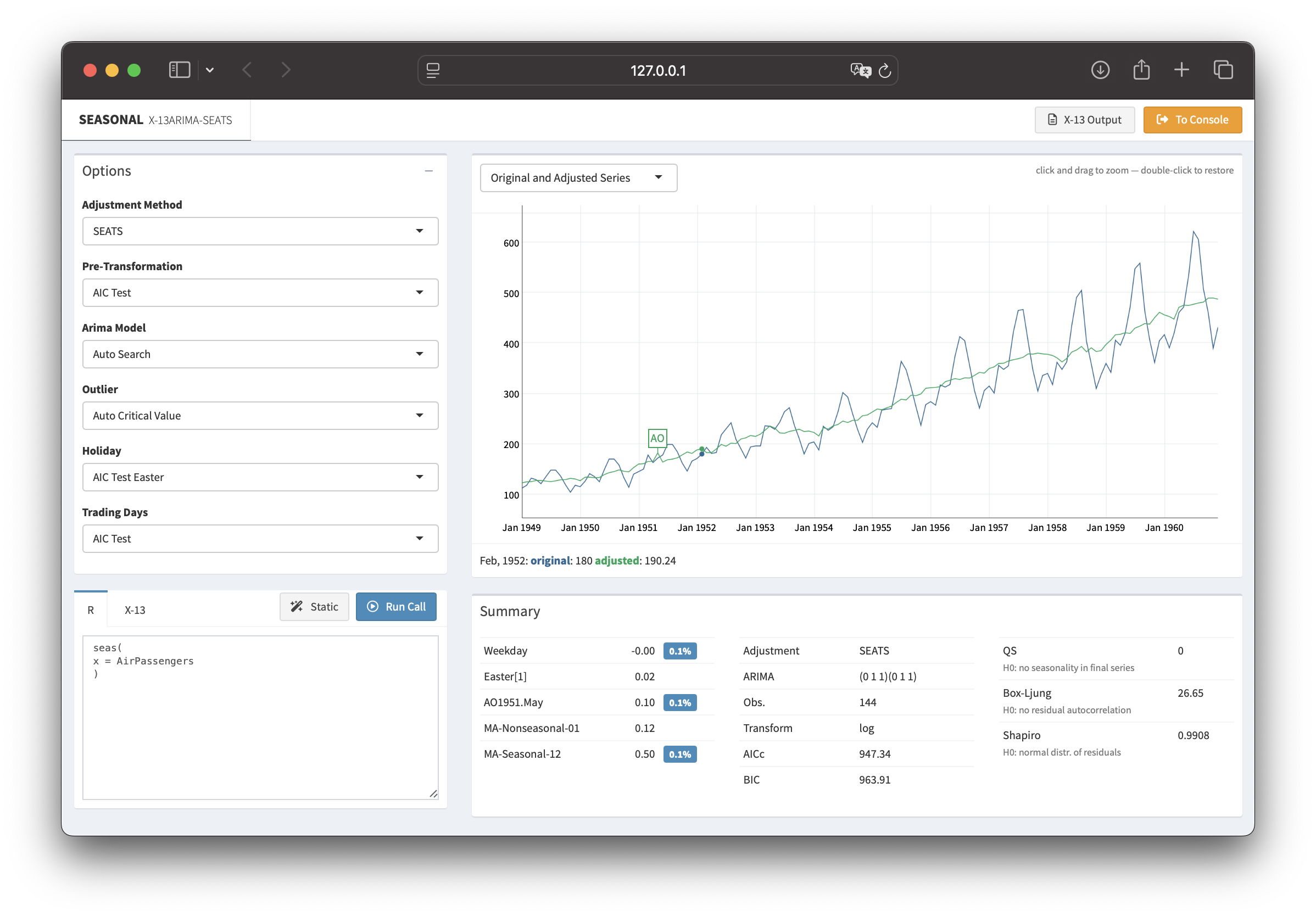
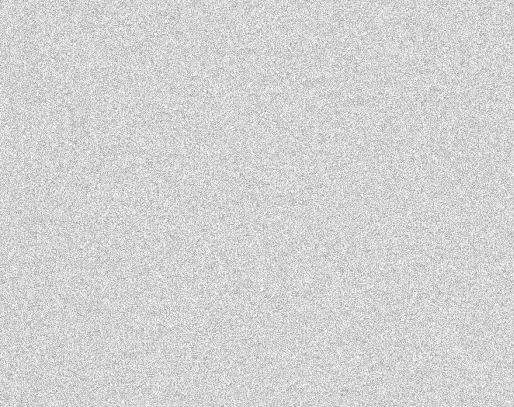
Christoph Sax /
seasonal 1.10: R-interface to X-13ARIMA-SEATS
time-series/R
Time series data often display recurring seasonal patterns throughout the year. The R package seasonal provides a powerful and user-friendly way to perform seasonal adjustment in R, leveraging the X-13ARIMA-SEATS procedure developed by the U.S. Census Bureau.
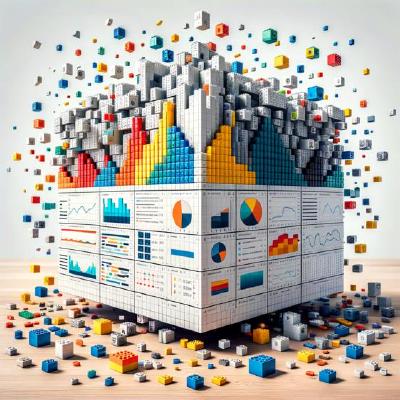
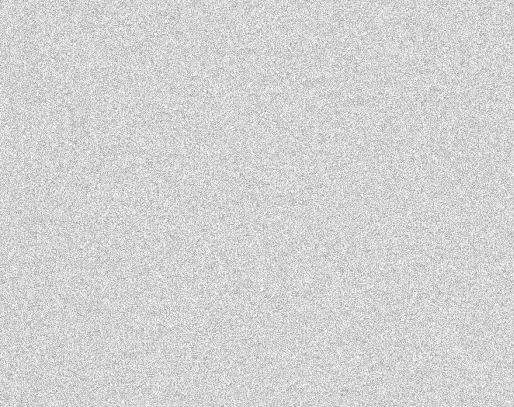
David Granjon /
Introducing blockr: a no-code dashboard builder for R
Shiny/R
Since 2023, BristolMyersSquibb, the Y company and cynkra have teamed up to develop a novel no-code solution for R. blockr is an R package designed to democratize data analysis by providing a flexible, intuitive, and code-free approach to building data pipelines.
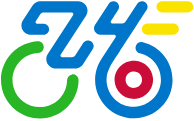
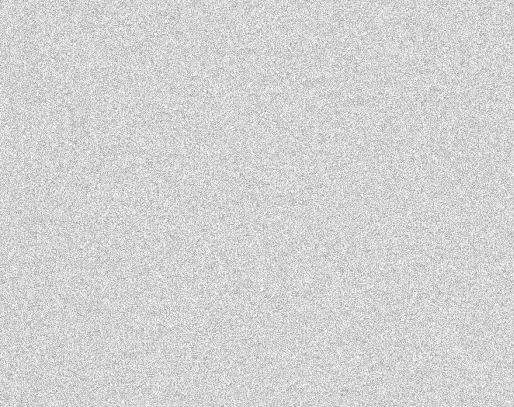
David Granjon /
2024 road and para-cycling road world championships: preliminaRy analysis
Sport/R
Analyzing the routes and elevation profiles of the 2024 road and para-cycling world championships in Zurich. This analysis uses R packages like gpx, leaflet, and rayshader to visualize and understand the challenges cyclists will face during the competition.
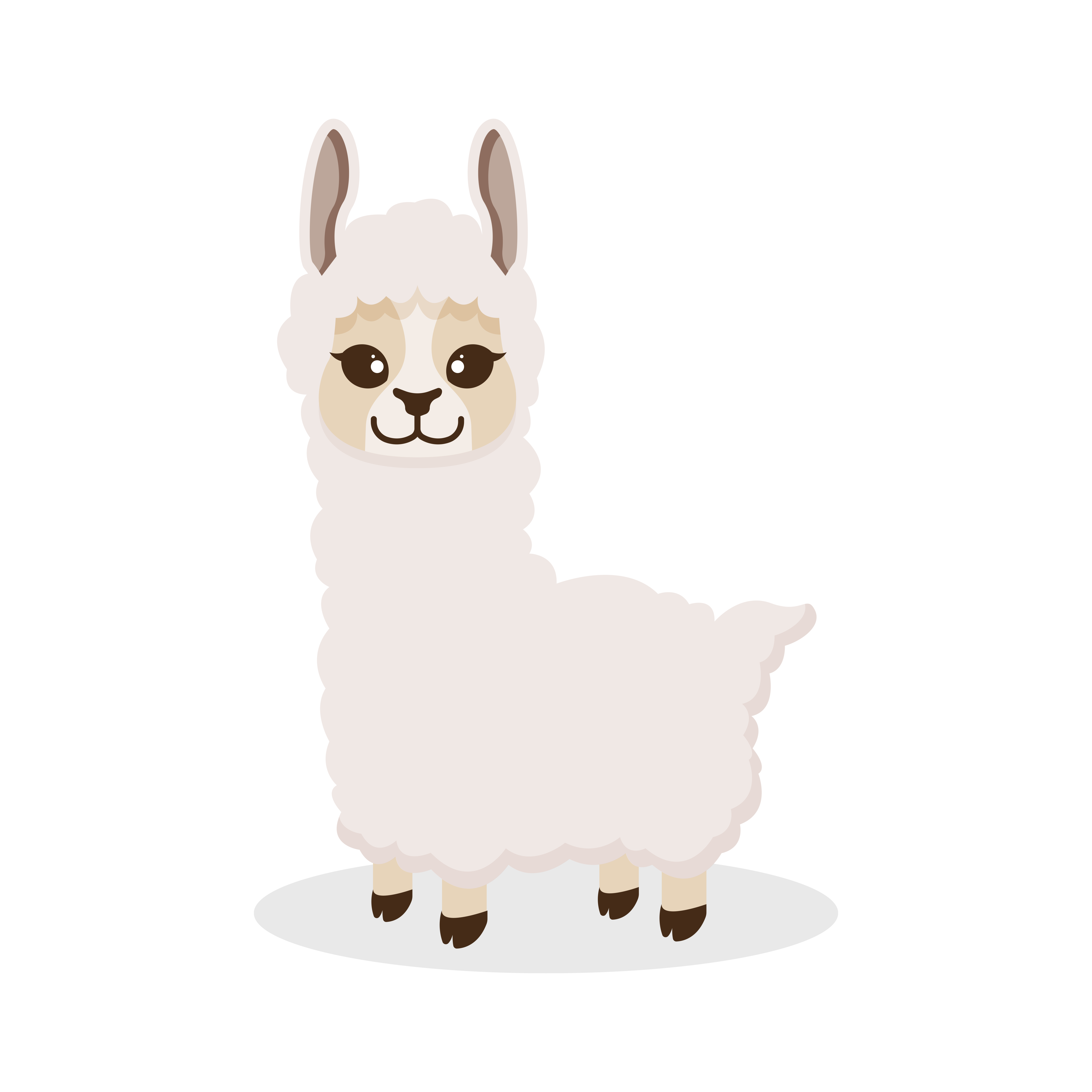
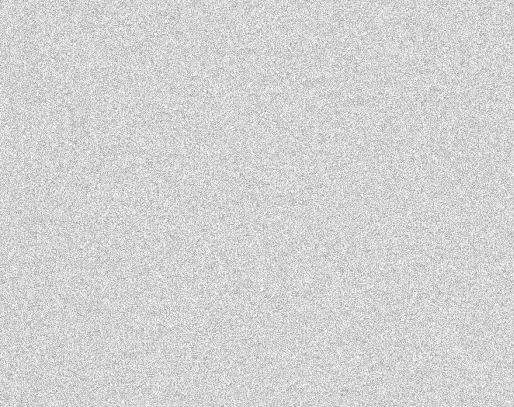
Christoph Sax /
Playing with Llama 3.1 in R
LLM
Meta recently announced Llama 3.1, and there's a lot of excitement. I finally had some time to experiment with locally run open-source models. The small 8B model, in particular, produces surprisingly useful output, with reasonable speed.
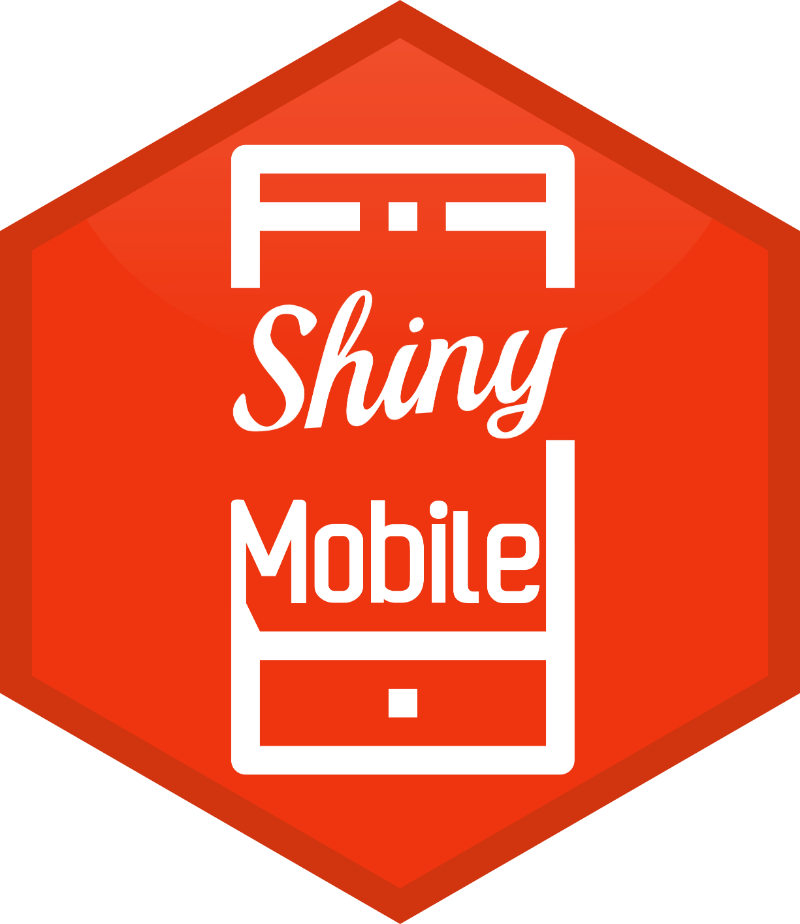
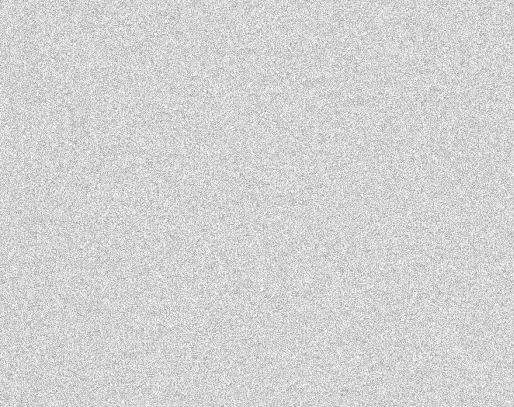
Veerle van Leemput, David Granjon /
shinyMobile 2.0.0: a preview
Shiny
shinyMobile has been enabling the creation of exceptional R Shiny apps for both iOS and Android for nearly five years. This year shinyMobile gets a major update to v2.2.0, bringing multi-page support, new components, and an upgrade to Framework7 v8.
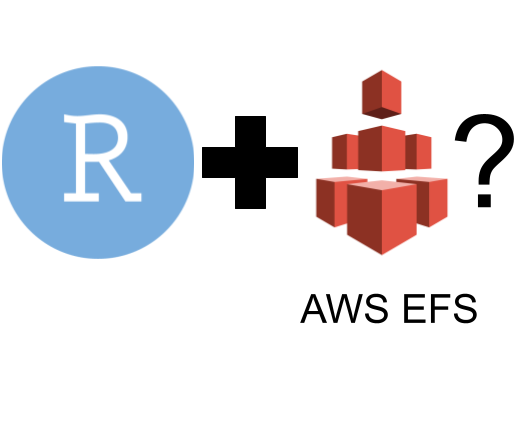
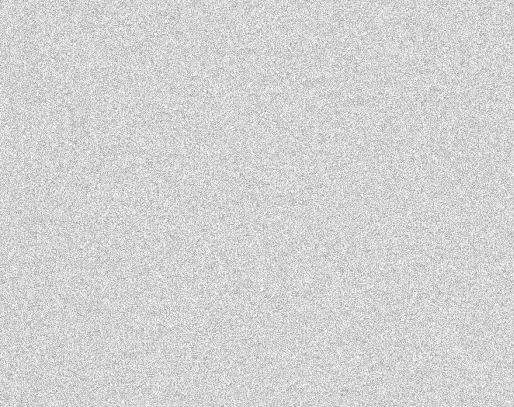
Patrick Schratz /
EFS vs. NFS for RStudio on Kubernetes (AWS): Configuration and considerations
DevOps/Posit/performance
As a consulting company with a strong focus on R and Posit (formerly RStudio) products, we have gathered quite a bit of experience in configuration and deployment of Workbench and Connect products in the cloud. We would like to share some of these insights and shine some light on the file system debate around these applications.
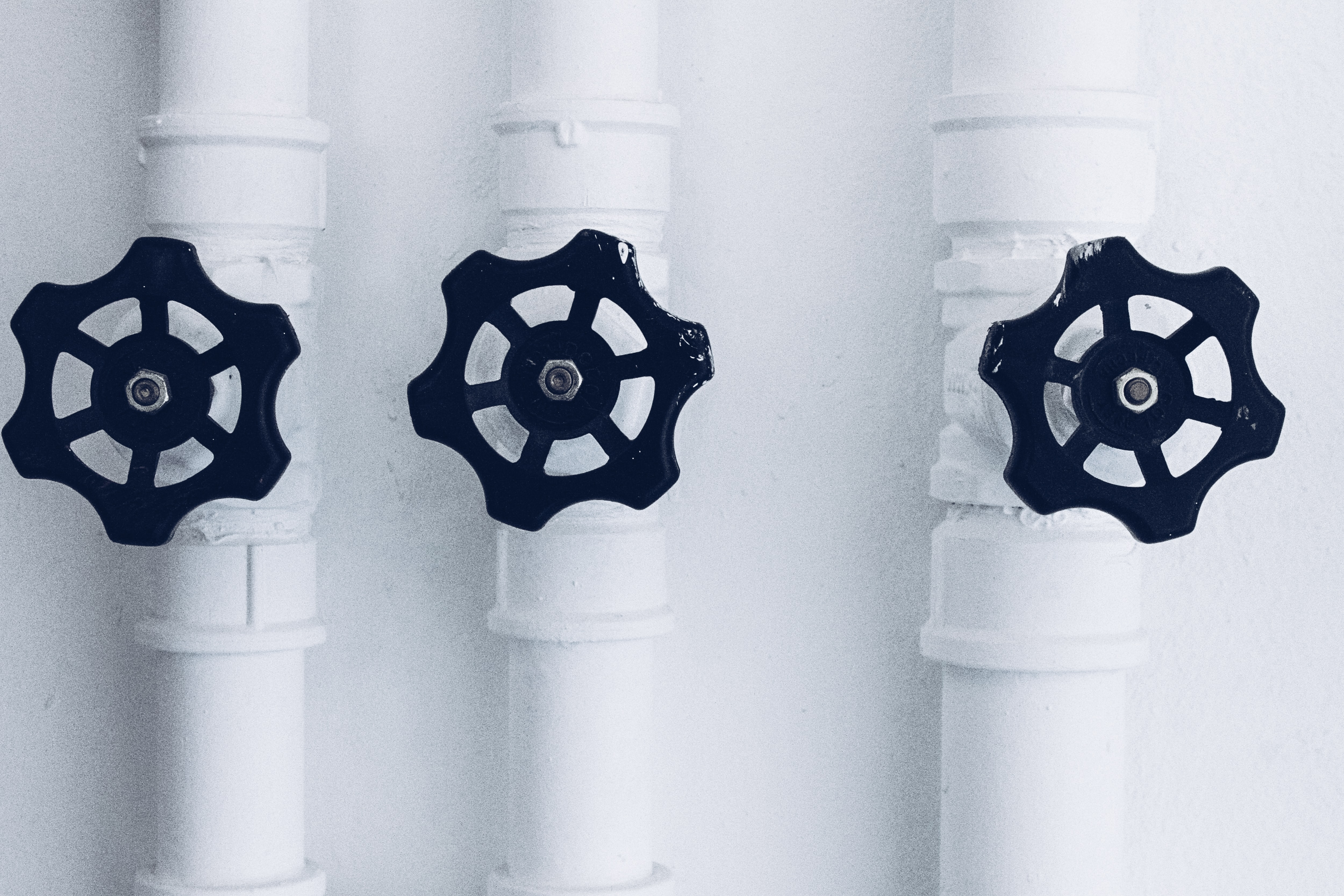
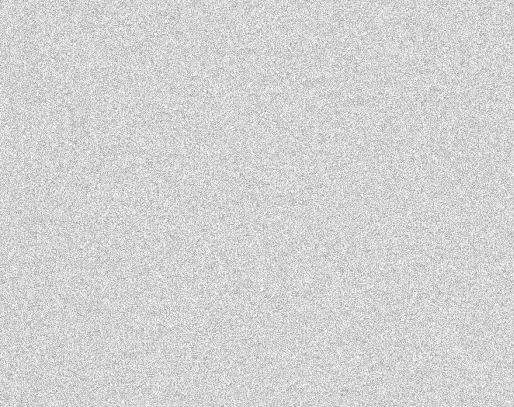
Patrick Schratz /
Accessing Google's API via OAuth2
DevOps
At cynkra we recently aimed to automate more parts of our internal toolstack. Google Workspace offers a comprehensive REST API which can be used for automation purposes. When interacting with an API, authentication is usually required.
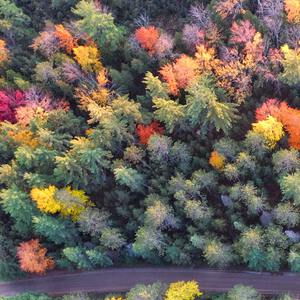
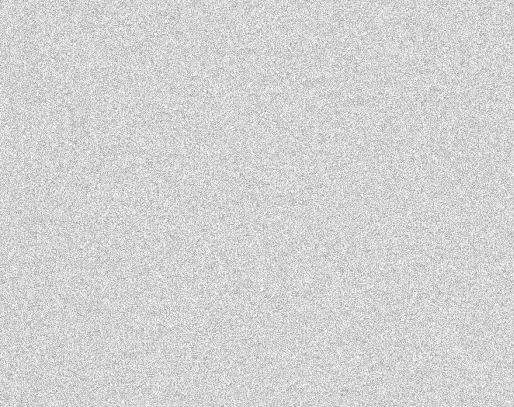
Christoph Sax /
seasonal 1.9: Accessing composite output
R/time-series
seasonal is an easy-to-use and full-featured R interface to X-13ARIMA-SEATS, the seasonal adjustment software developed by the United States Census Bureau. The latest CRAN version of seasonal fixes several bugs and makes it easier to access output from multiple objects.
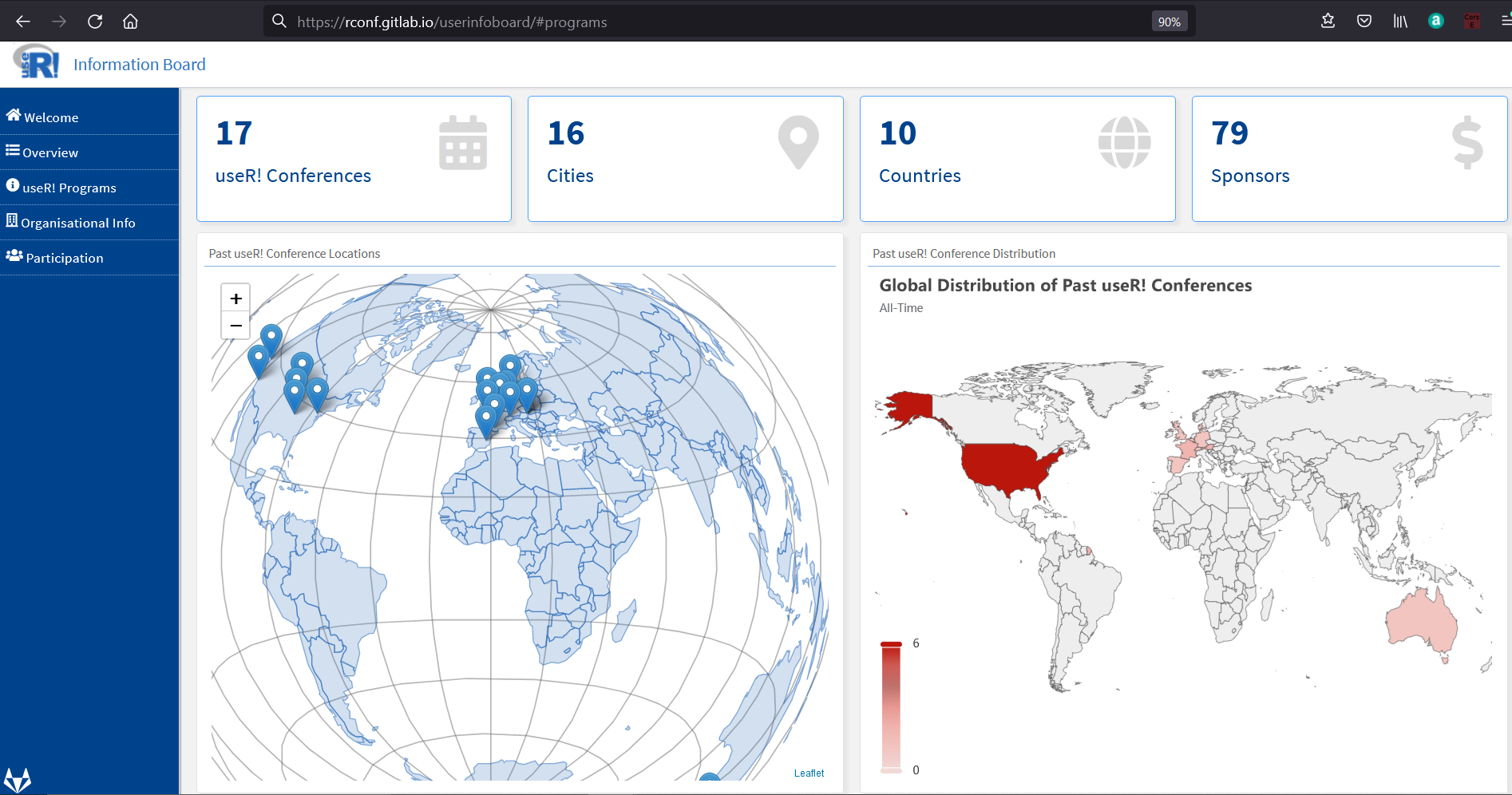
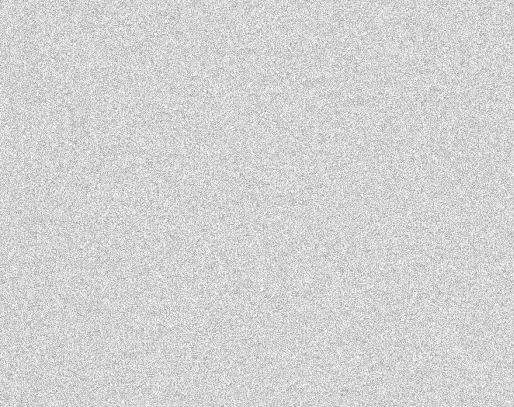
Ben Ubah /
Google Season of Docs with R: useR! Information Board
R
Google Season of Docs provides support for open source projects to improve their documentation and gives professional technical writers an opportunity to gain experience in open source. The R Project participated in GSoD as an open-source organization for the first time this year.
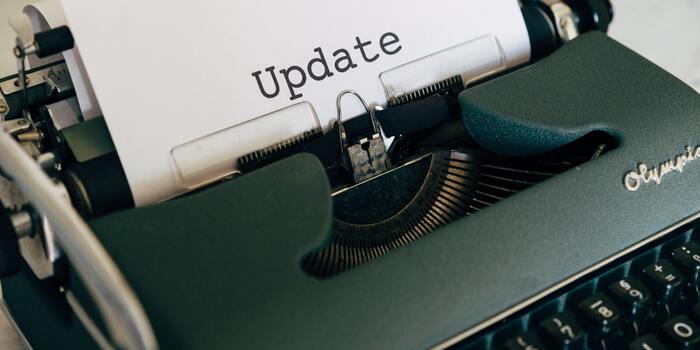
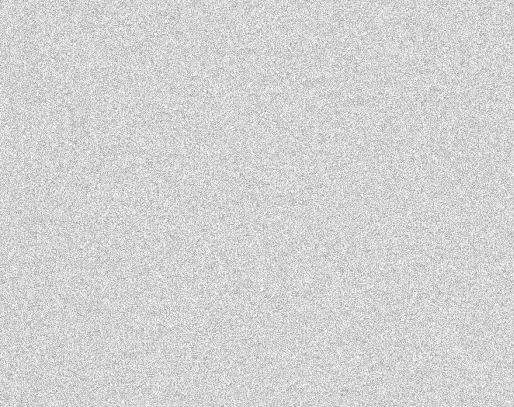
Kirill Müller /
Running old versions of TeXlive with tinytex
R
Rendering PDFs with rmarkdown requires a working LaTeX installation, such as tinytex. Occasionally, existing workflows break with the newest version of LaTeX. This post describes how to run an older LaTeX version for just a little while.
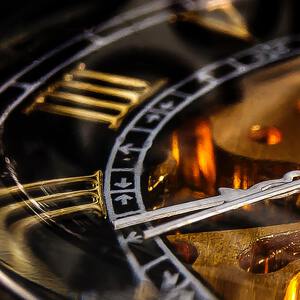
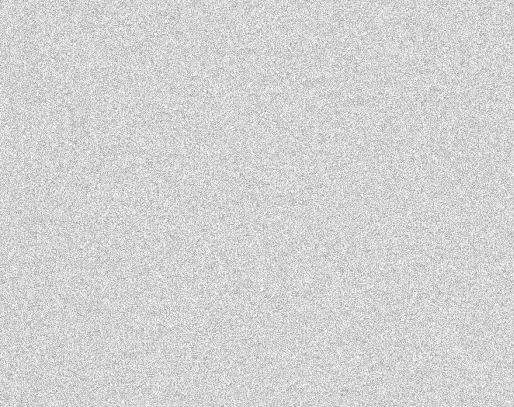
Christoph Sax /
tsbox 0.3.1: extended functionality
R/time-series
The tsbox package provides a set of tools that are agnostic towards existing time series classes. The tools also allow you to handle time series as plain data frames, thus making it easy to deal with time series in a dplyr or data.table workflow.
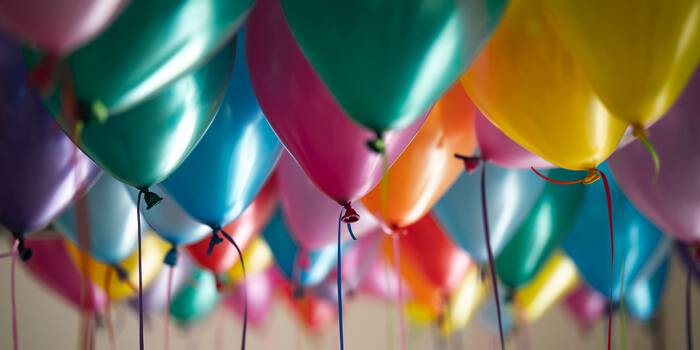
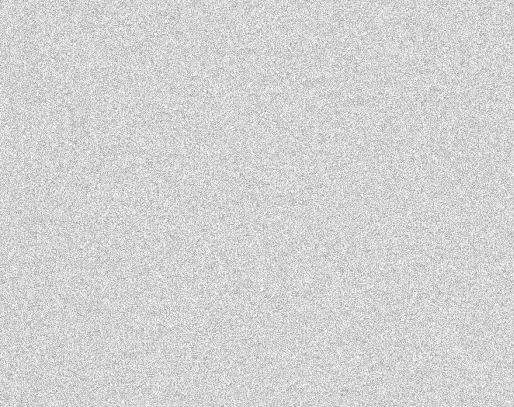
Cosima Meyer, Patrick Schratz /
Celebrating one-year anniversary as RStudio Full Service Certified Partner
Posit/DevOps
cynkra celebrates its first anniversary as an RStudio Full Service Certified Partner! Every day, we help our clients set up professional IT infrastructures using RStudio products and license compositions suited to their individual needs.
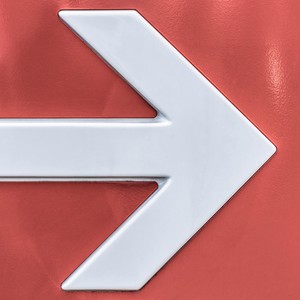
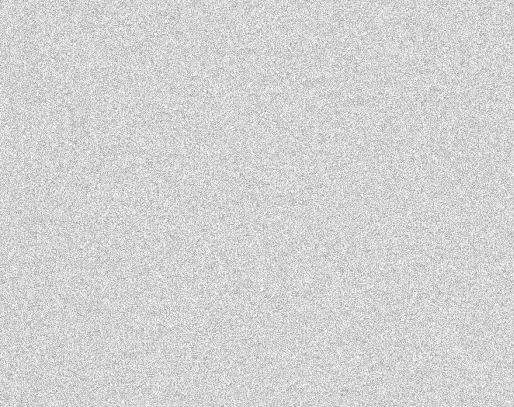
Patrick Schratz, Kirill Müller /
Deprecating a pkgdown site served via GitHub Pages
R
Websites for R packages built with pkgdown have become a de-facto standard over the last few years. Sometimes, repositories are transferred to a new user/organization or the package is renamed. This post proposes several ways to handle pkgdown URL redirection gracefully.
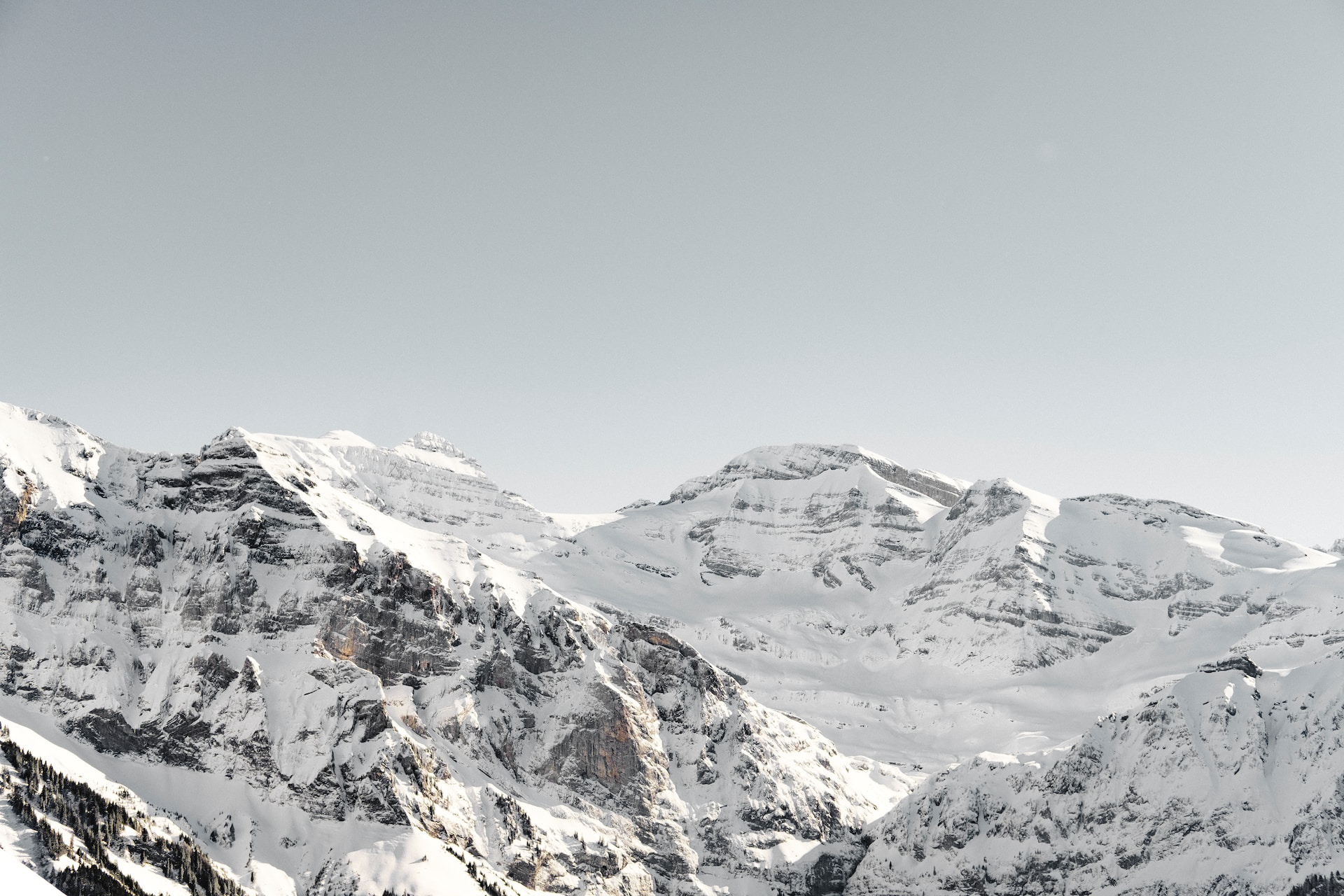
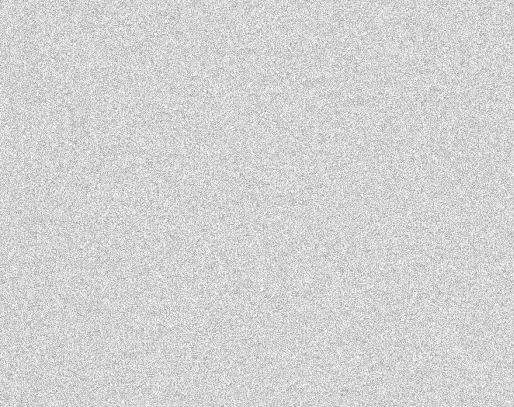
Patrick Schratz /
gfortran support for R on macOS
R/DevOps
For a long time, gfortran support on macOS could be achieved by installing the homebrew cask gfortran. As of 2021, both the brew cask command and the cask gfortran are deprecated. This article explains how to set up gfortran for R package development on macOS.
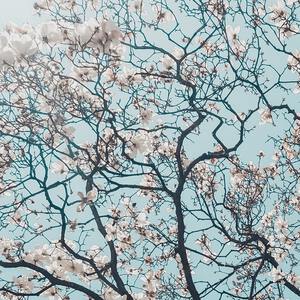
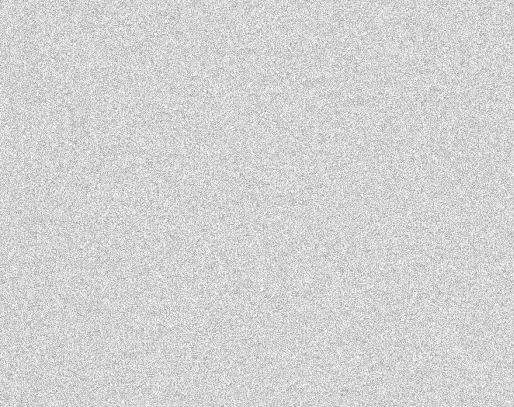
Christoph Sax /
Seasonal Adjustment of Multiple Series
R/time-series
seasonal is an easy-to-use and full-featured R-interface to X-13ARIMA-SEATS, the seasonal adjustment software developed by the United States Census Bureau. The latest CRAN version of seasonal makes it much easier to adjust multiple time series.
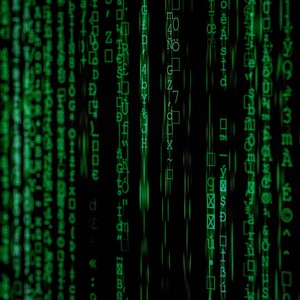
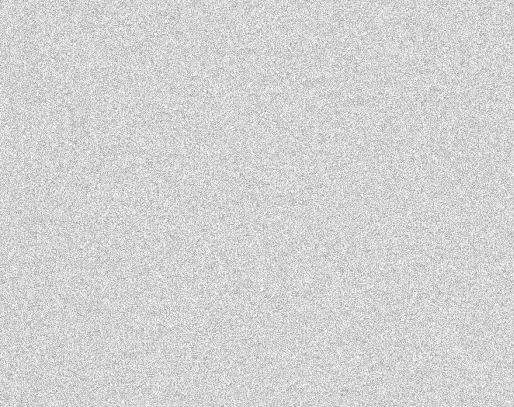
Kirill Müller /
Dynamic build matrix in GitHub Actions
R/DevOps
GitHub Actions allows automating build and deployment processes (CI/CD), tightly integrated with GitHub. A build matrix is a way to define similar workflows that differ only by configuration parameters. This post shows how to define these build matrices dynamically.
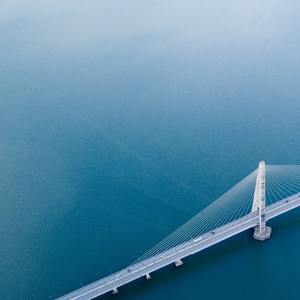
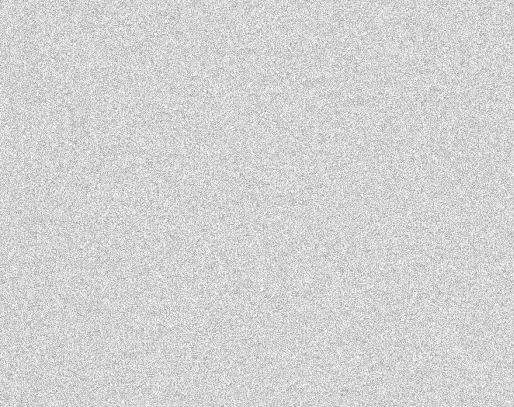
Patrick Schratz /
Setting up a load-balanced Jitsi Meet instance
DevOps
Jitsi Meet is a self-hosted Free and Open-Source Software (FOSS) video conferencing solution. During the recent COVID-19 pandemic, the project became quite popular, and many companies decided to host their own Jitsi instance.
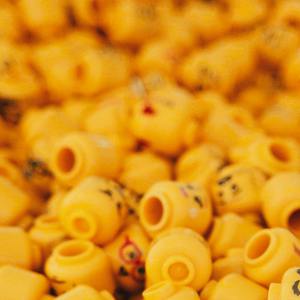
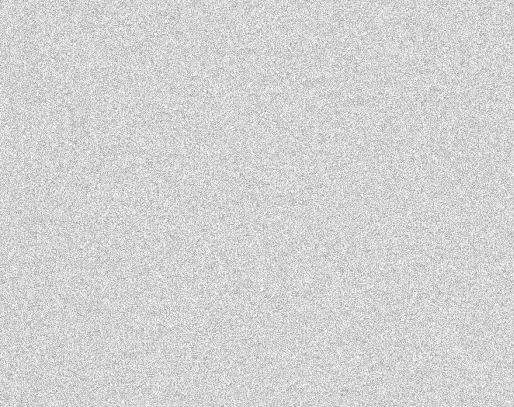
Kirill Müller /
Maintaining multiple identities with Git
DevOps
When committing to a Git repository related to my consulting work, I must use my company e-mail address. Not so much for my open-source work where I prefer to use other e-mail addresses. Having to configure the e-mail address for each repository separately eventually gets annoying.
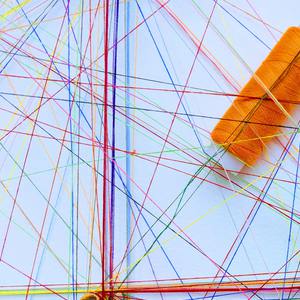
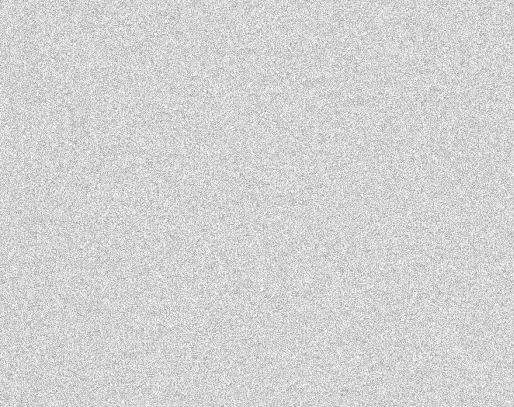
Angel D'az, Kirill Müller /
Relational data models in R
R/databases
Relational databases are powerful tools for analyzing and manipulating data. However, many modeling workflows require a great deal of time and effort to wrangle data from databases to place it into a flat data frame or table format. The dm package takes the advantage of relational modeling and brings it to R.
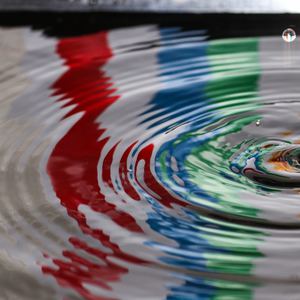
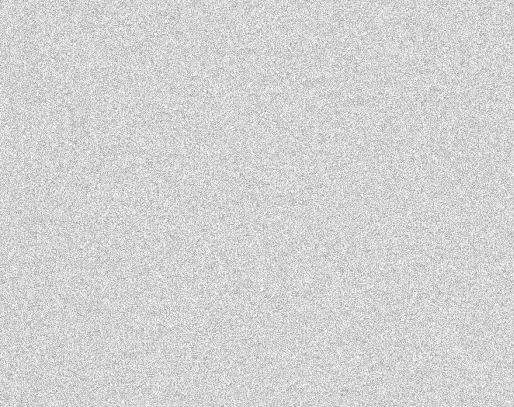
Christoph Sax /
tempdisagg: converting quarterly time series to daily
R/time-series
Not having a time series at the desired frequency is a common problem for researchers and analysts. With the help of one or more high-frequency indicator series, the low-frequency series may be disaggregated into a high-frequency series.
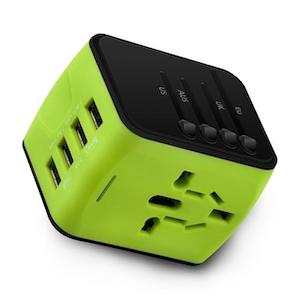
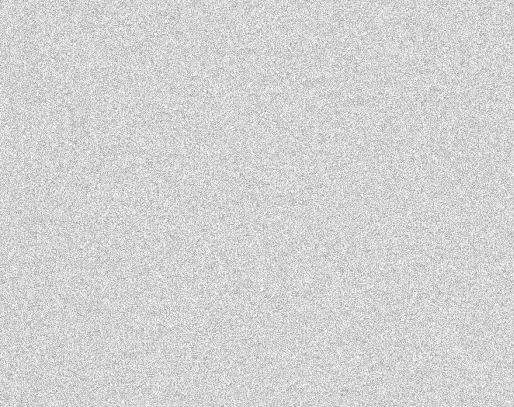
Christoph Sax /
tsbox 0.2: supporting additional time series classes
R/time-series
The tsbox package makes life with time series in R easier. It is built around a set of functions that convert time series of different classes to each other. They are frequency-agnostic and allow the user to combine time series of multiple non-standard and irregular frequencies.
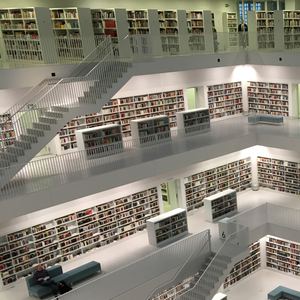
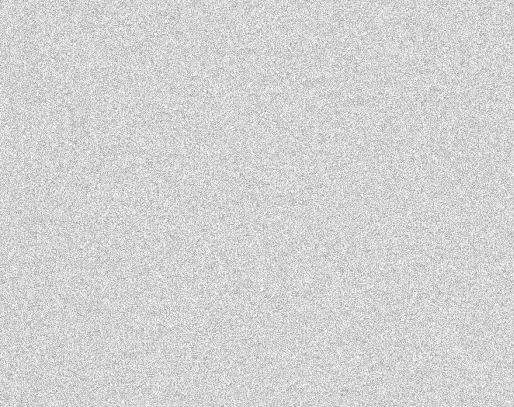
Balthasar Sager /
Introducing dm: easy juggling of tables and relations
R/databases
The dm package reduces your mental strain when working with many tables. It connects your data, while still keeping them in their original tables. You can easily perform operations on all tables, visualize how they're connected, and integrate databases.
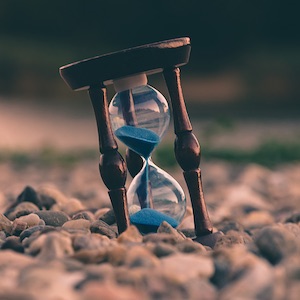
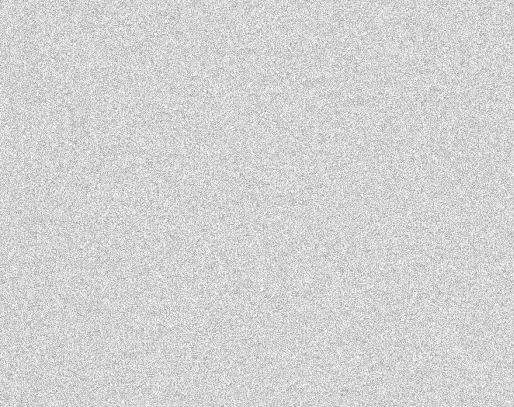
Christoph Sax /
tsbox 0.1: class-agnostic time series
R/time-series
The R ecosystem knows a vast number of time series classes: ts, xts, zoo, tsibble, tibbletime or timeSeries. The plethora of standards causes confusion. tsbox provides a set of tools that make it easy to switch between these classes.
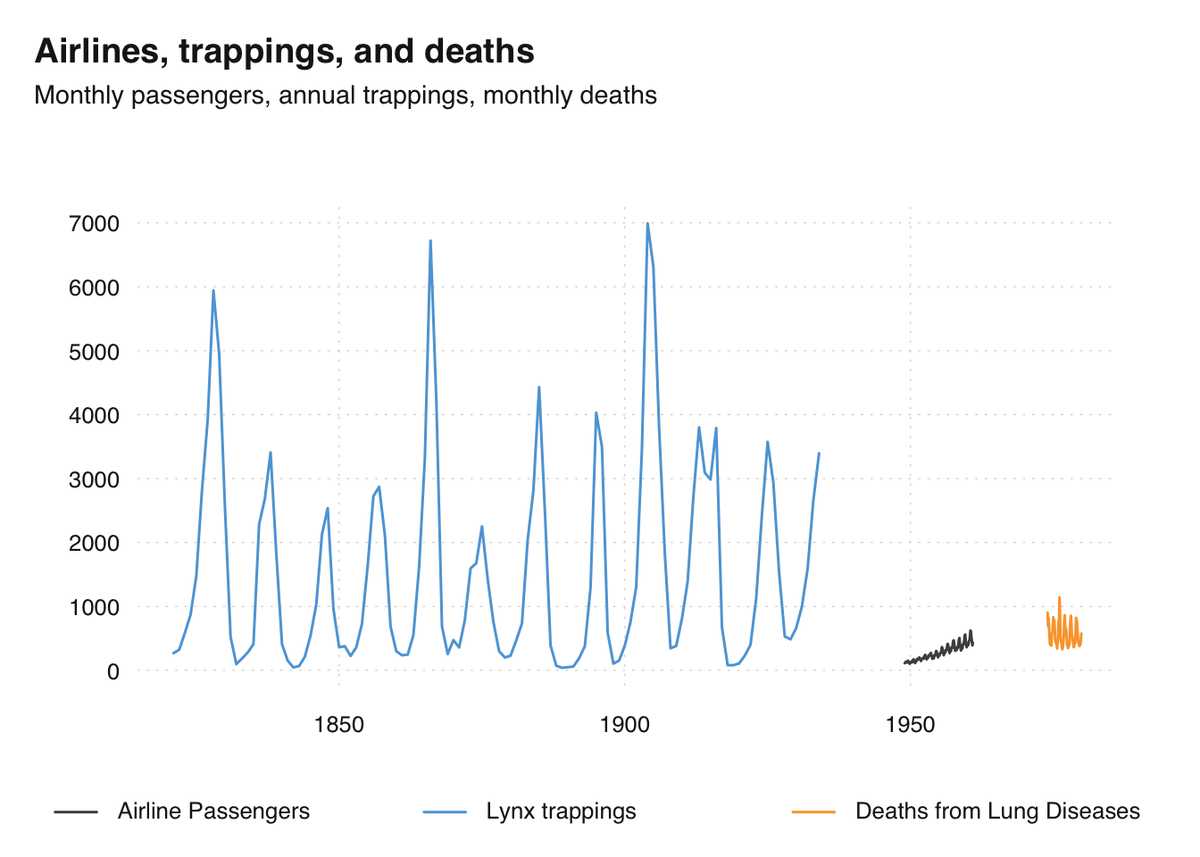
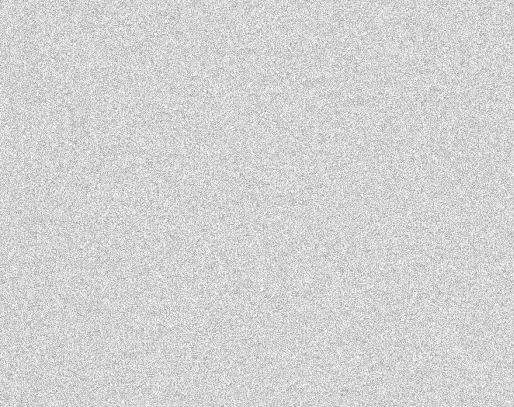
Christoph Sax /
Time series of the world, unite!
R/time-series
The R ecosystem knows a ridiculous number of time series classes. tsbox provides a set of tools that are agnostic towards existing time series classes, built around converters that transform between different time series formats.
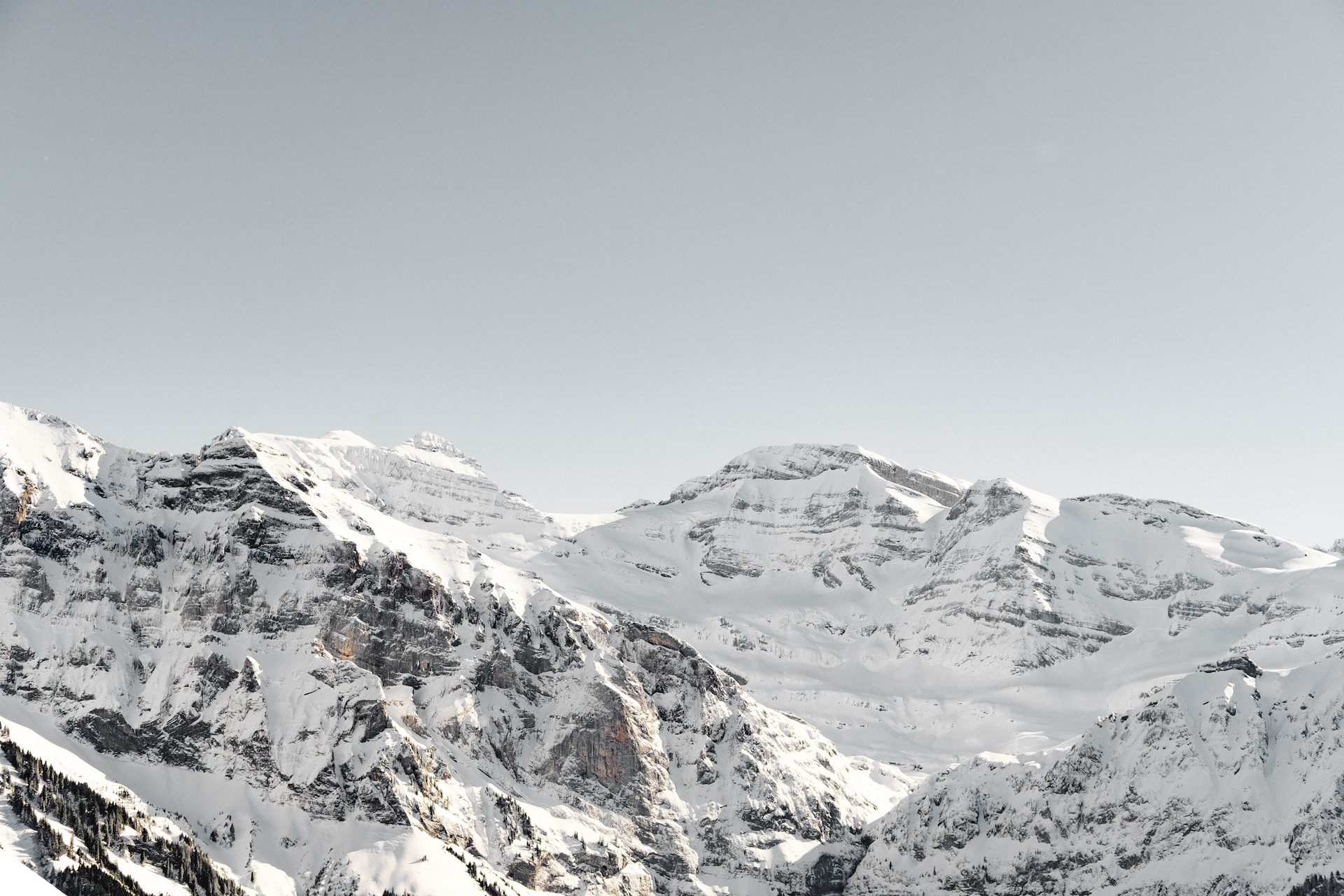
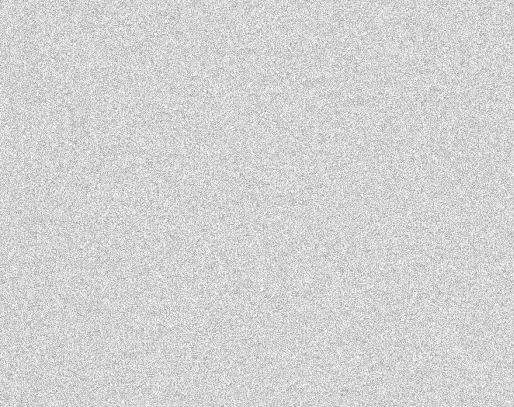
Kirill Müller /
Done “Establishing DBI“!?
R/databases
The “Establishing DBI“ project, funded by the R consortium, started about a year ago. It includes the completion of two new backends, RPostgres and RMariaDB, and quite a few interface extensions and specifications.